Charles V. Trappey(*1)
Department of Management Science National Chiao Tung University Hsinchu, Taiwan
Amy J.C. Trappey(*2)
Department Industrial Engineering and Engineering Management National Tsing Hua University Hsinchu, Taiwan
*1 Prof. Charles V. Trappey is with the Department of Management Science, National Chiao Tung University, Hsinchu, Taiwan (e-mail: trappey@faculty.nctu.edu.tw). Charles is on the Editorial Board of Psychology and Marketing. He is an Australian Trademark Attorney.
*2 Prof. Amy J.C. Trappey is with the Department of Industrial Engineering and Engineering Management, National Tsing Hua University, Hsinchu, Taiwan (e-mail: trappey@ie.nthu.edu.tw). Amy serves as Associate Editor for Advanced Engineering Informatics, IEEE Trans. SMC: Systems, and World Patent Information. She is the Fellow of ASME, ISEAM, and CIIE.
Abstract
Consumer and brand loyalty related patents and non-patent literature (NPL) are surveyed over a twenty-year period. The first goal of the study is to determine the domain boundaries of research and development (R&D) for enforcing ethical consumer and brand loyalty programs. After analyzing the domain ontology of the consumer and brand loyalty, the second goal of the study is to disclose how the loyalty systems can be corrupted by unethical practices, especially in the food supply chain. The collective intelligence of patents and NPL identifies the key innovative R&D topics, their technical components, and viable applications that enhance brand and consumer loyalty. Patents and NPL are mined using the natural language processing machine learning approach to discover the semantic distribution and clustering of key technologies. This research discovers four clusters of topics over the past twenty years. The research systematically analyzes how these applications, intended to enhance food quality and safety through brand loyalty and consumer trust, could be exploited and disrupted by unethical competitors or entities. These disruptions, such as distorting the values of trademarks, misleading the source of food supplies, and other types of unethical behavior in the omni-retail food channels, can be used to form effective strategies to ensure trust and safety in food supply chain.
Keywords
Brand and consumer loyalty, unethical channel behavior, food supply chain, AI, machine learning
1 Introduction
In this section, machine learning (ML) is used to perform semantic analysis of patents and NPL publications over a twenty-year period. The method uses the R&D collective intelligence created over this time period to create consumer and brand loyalty solutions. Three main text mining methods applied in this research are reviewed in Section 2. The ontology map of the mainstream topics (clusters) and the key terms of each topic discovered are described in Section 3. Section 4 demonstrates how these systems can be corrupted through unethical means to disrupt the global food supply chain and consumer brand loyalty, damaging the trust and brand equity of food suppliers and services.
Our research explores the recent topics and trends of technologies and applications used for enhancing brand and consumer loyalty. The methodology includes a comprehensive literature and patent document collection and review from a pay-for-use search engine. A total of 910 documents (605 patents and 305 NPL articles) are collected using the InnovationQ Plus search engine (InnovationQ 2020). These articles form the document corpus for e-discovery of the leading brand and consumer loyalty R&D topics and trends. This research uses unsupervised machine learning approaches to text mine and extract the key topics and key terms (including words and phrases). The Latent Dirichlet Allocation (LDA) algorithm is used for topic modeling, K-means for technology clustering, and normalized term frequency-inverse document frequency (NTF-IDF) for key term discovery. The results generate a comprehensive overview and ontology schema for further depiction of unethical (or even illegal) acts and risks affiliated with these brand and consumer loyalty solutions. Ethical in the sense that they may be legal but also may be intentionally misused, especially in the global food network.
2 Literature Review
This section reviews past research literature related to brand and consumer loyalty and AI machine learning. A collection of articles and patents related to brand loyalty are collected as a corpus for analytic research and review over the past twenty years. The research trends and methods related to brand and consumer loyalty are organized so that an ontology can be constructed to better understand the clusters of approaches used and the main node and relationships among techniques over time. The numbers of patents and literature (NPLs) published from 2000 to 2019 are collected for review and analysis. The patents are collected from global patent offices and the literature are from major global online libraries: IEEE Xplore Publications, PubMed Central, an online archive of journal literature in biomedical and life sciences, and the IP.com journal documenting new disclosures that have never been published anywhere else in the world. The multi-organizational library comes from 20 publishing partners who provide access to over 180,000 items with new content being added weekly (InnovationQ 2020). In Section 2.2, we will briefly review the literature about AI and machine learning (ML) (Alpaydin 2009), which will be adopted as Section 3’s document content analysis methodology.
2.1 Brand and Consumer Loyalty
E-commerce has changed retail business models where mobile devices and social networks underlie retail innovations and enable data transfer and communications between buyers, sellers, and supply chain intermediaries. Many suppliers or intermediaries have built omni-channels. The concept of omni-channel distribution and logistics is to integrate online and offline resources to provide versatile and satisfactory shopping experiences for customers (Trappey et al. 2017). Retail brand and customer loyalty has changed over time since omni-channel (online and offline) sales are linked to social, legal, economic, government policy, and technological standards. For example, emerging technologies and standards for the Internet-of-Things (IoT) enables solutions supporting smart logistic services and smart payment methods for state-of-art omni-channel e-commerce (Trappey et al. 2017). Due to the massive growth of commerce through omni-channels, means to enhance brand and customer loyalties becomes critical to minimize the un-ethical conduct of buyers, sellers and intermediary players during the transactions. The most common case example is to promote a company’s brand awareness and its consumer loyalty by affiliating the brand with the company’s high-quality goods and services. Thus, the un-ethical act of purposely confusing consumers with fake goods (labeled using a similar brand-name) can be prevented through the application of new technologies (e.g., QR code or embedded chip) or laws (e.g., registered TM or patents).
Global consumer food demand requires that most stores include physical locations, virtual channels and e-commerce sales systems. A company will lose competitive advantage if it cannot create customers that are loyal to both the store’s internet-based system and the physical store. Omni-channels were introduced in the literature around 2007 to enable businesses to interact with consumers through multiple channels rather than a single point of contact. After the academic research matured, companies began applying for patents around the year 2011. The omni-channel concept has evolved from single-channels, multi-channels, and cross channels (Yan 2014).
The greatest difference between omni-channel sales and traditional sales is that the former integrates the services and resources of more than two sales channels. Combining the sales data using intelligent methods, sellers can analyze the behavior of customers and develop an appropriate sales strategy to achieve consistent shopping experiences with high satisfaction for customers. To accomplish this task, sellers must provide a variety of logistics storage and distribution methods that satisfy the customers’ service and delivery demands (Verhoef et al. 2015; Brynjolfsson et al. 2009; Beck and Rygl 2015; Khan and Greaves 2008). Channels which are co-dependent provide a flexible and seamless shopping experience for both online or offline customers. Omni-channel sales use logistics models, cash flows, and information flows for both domestic and cross-border sales. The application of strategic analysis and planning provides a smart, integrated logistic model, and system. These omni-channel systems, however, are not risk free and can cause the destruction or boycott of a food brand and the loss of a large group of customers almost instantaneously. Such practices are being coming more frequent in the news, without verification and without proof especially if politically motivated. Examples include the boycott of Chick-Fil-A franchises (Lucas 2020) and most recently Goya canned good products (Nguyen 2020).
The social environment over the last twenty years has significantly changed the behavior of consumers. Innovations such as social networking and the ubiquitous internet has greatly enhanced e-commerce popularity. The models of consumer behavior using omni-purchasing channels are quite different from the traditional retail sales approach. In order to improve the social environment for omni-channel electronic commerce activities, environmental changes are needed. For instance, a cloud sharing system can provide dynamic trading information between suppliers, customers and intermediaries. Data mining and analytical approaches are used to model the changing demands. These data assist suppliers to rapidly dispatch and fulfill orders for all channels using socially accepted processes. These processes can also become grey channels if the true supplier of food and services do not continuously monitor the internet for infringement or other trade abuse.
With the development of O2O (online to offline), and O2M (online to mobile), companies integrate virtual and physical sales channels to maintain customer loyalty. Governments must pass relevant laws to provide a regulated and protected environment that allows fair and ethical operation of omni-channel sales and distribution across the global environment. For example, governments created intellectual property laws to protect IP rights and avoid infringement of copyrights, trademarks, patents, business processes, and designs during commercial operations. Firms that introduce new technology to build an exclusive omni-channel model require IP law protection. Governments also regulate the return and replacement of goods and legislate laws to prevent customers from entering into sales channel disputes.
Changes in the economic environment require companies to continually re-evaluate their competitive strengths and their business processes. Companies that fail to re-configure their business processes lose strategic competitive advantage and are faced with the inability to better manage inventories and lower costs. Food retailers connect with new customers through many channels. These channels are also known as touch points and include internet sales. Retailers experiencing low sales growth via omni-channels are facing operational inefficiencies that increase costs and lower profit margins.
The policy environment for omni-channels and smart logistics create the foundation for governments to promote national economic growth (Beck and Rygl 2015). China, with a large land mass and a widely dispersed population centers, emphasizes the integration of consumer shopping behavior through internet sites, social media, mobile devices, and online advertisements. Taobao and Alibaba are leading industries that have created a retail revolution across China by implementing omni-channels. Industry 4.0 was promoted by the Germany government in 2011 as a means to create and stimulate the use of smart factories. The implementation of these technologies (Prime Policy of Japan, 2013) requires horizontal integration of the value network, vertical integration of the manufacturing network, and communication linkages with workstations and cyber-physical systems (CPS). The 2013 Japanese industry revitalization plan was proposed to facility investment in R&D for equipment to revitalize manufacturing and retail businesses. The objective was to improve the interaction between machines and improve supply chain flows. By developing new sensors, control and drive systems that use cloud computing and artificial intelligence, the internet linked robots have become the core of omni-channel smart logistic system development. Productivity 4.0 was proposed in 2015 by Taiwan government (Executive Yuan 2015). The policy emphasizes applications of new information and communication technologies for networking, cloud computing, big data analysis and the creation of artificial intelligence for domestic and global value chains. Food suppliers, distributors, and retailers can use big data, IoT, and cloud services to facilitate the development of omni-channels.
The technical environment and standards play an important role to enable collaboration, integration, and inter-changeability in technology environment. Some key technologies are cloud system that enables Internet of Things (IoT) technology to monitor and exchange product and sales information. Consumer behavior is better monitored in real-time and demand, preferences, attitudes, and opinions help create a more competitive environment. The voice of the customer is clearly heard and quick adaptations to products and preferences can be made to enhance consumer loyalty to the brand (Trappey et al. 2017). The omni-channel data collected via RFID or IoT devices are modeled and analyzed using big data analytics. The analyses, then, yield insights to predict consumer demands. The results help food retailers and supply chain modify marketing strategies and provide greater customization.
2.2 Artificial Intelligence
The development of AI can be traced back to 1940’s. AI covers any techniques which enable computers to behave like a human (Russell and Norvig 1994). In other words, any intelligence demonstrated by a machine that leads to an optimal solution can be included as an AI application. Since the 1980s, the concept of Machine Learning (ML) started to be increasingly applied to analyze trends and form large scale searches. ML learns from past data and experiences to find rules for optimal behavior and decision making. Over the past decade, Deep Learning (DL) has become the replacement technology and a research mainstream in artificial intelligence. Inspired by the function and systems of the brain, DL can learn data patterns using multi-layers of neural networks.
The new methodologies of AI have been applied to a vast range of fields including engineering, medicine, finance, and driving. All aspects of human lives are moving towards the inclusion of intelligence enhanced applications. For this ubiquitous process to succeed, cloud computing, big data, and DL capabilities are indispensable. The development paths of AI, ML, and DL over a timeline from the 1950’s to the year 2010 and further are described in (Li et al. 2019). This research has adopted AI machine learning algorithms for systematic reviews and analyses of patents and NPL publications to discover the R&D trends in consumer and brand loyalty solutions.
3 Methodology
The first step of the research is to determine the domain boundaries of research and development trends of ethical brand and customer loyalty programs by analyzing the knowledge, methodologies, and system developments disclosed in the related patents and NPL publications. The second step is to investigate how these systems can be corrupted in the food supply chain by unethical practices. Total of 305 papers and 605 patents relating to brand and consumer loyalty between the years 2000 and 2019 are collected. The abstracts and introductions of these publications are used to analyzed the frequency and importance of terms and phrases for the domain ontology construct. The text mining methods, including LDA, K-means, and key term extraction (NTF-IDF), are adopted and cross-referenced for e-discovery of the main technical topics and sub-topics defined by key terminologies.
4 Topic Clusters of Consumer and Brand Loyalty Discovered
Using the LDA probabilistic-based topic modeling technique and the k-mean clustering verification, the consumer and brand loyalty ontology has been defined and categorized in four clusters with respective topic interpretation (Hartigan and Wong 1979). Cluster 1 focuses on “Customer Transaction Business Intelligence (BI) Analysis”. Table 1 highlights the cluster key terms. The use of transaction-based loyalty services enables merchants to provide personalized recommendations or reward program to attract customers to return and increases customers' trust and loyalty to the brand.
Table 1: Top 10 key phrases of Cluster 1 - Customer transaction BI analysis.
Key phrase | Probability | Key phrase | Probability |
transaction information | 0.1060 | member loyalty | 0.0594 |
loyalty card | 0.0892 | purchase transaction | 0.0560 |
card issuer | 0.0843 | loyalty account | 0.0538 |
reward program | 0.0820 | service provider | 0.0506 |
loyalty reward | 0.0715 | loyalty benefit | 0.0495 |
Cluster 2, Device and Platform Apps and User Interfaces, covers the topics of online applications (apps) and their user interfaces (UIs). Many documents in cluster 2 discuss systems or medium related to users, such as social networking systems, mobile applications, websites, and messaging functions as listed in Table 2. These systems collect and analyze the user’s profile in order to improve online service with well as improve the user experience.
Table 2: Top 10 key phrases of cluster 2 - Device/platform applications and user interfaces.
Key phrase | Probability | Key phrase | Probability |
user account | 0.1305 | digital content | 0.0527 |
social medium | 0.0947 | user profile | 0.0505 |
user interface | 0.0824 | customer payment | 0.0484 |
mobile application | 0.0712 | alphanumeric sequence | 0.0442 |
web site | 0.0604 | real time | 0.0428 |
In Table 3, the top key terms of Cluster 3 indicate that the main topic is Customer Relationship Management (CRM). In cluster 3, patents and NPL mostly deal with tracking customer satisfaction and loyalty information.
Table 3: Top 10 key phrases of cluster 3 - Customer relationship management (CRM).
Key phrase | Probability | Key phrase | Probability |
customer loyalty | 0.5462 | customer relationship | 0.0765 |
customer satisfaction | 0.2375 | relationship quality | 0.0660 |
relationship management | 0.1045 | customer equity | 0.0650 |
service quality | 0.0825 | customer engagement | 0.0550 |
customer database | 0.0765 | customer value | 0.0550 |
Cluster 4 is relevant to brand equity assessment. Brand equity refers to the perceived value of ethical brand and consumer loyalty, established brand names, and logos. This is a crucial concept in the field of brand management and marketing. The measures of brand equity are composed of factors indicating customer’s preferences. Table 4 shows the key terms appealed in the related publications.
Table 4: Top 10 key phrases of cluster 4 - Brand equity evaluation.
Key phrase | Probability | Key phrase | Probability |
brand equity | 0.3497 | famous brand | 0.1166 |
brand community | 0.2616 | consumer brand | 0.1072 |
social medium | 0.1768 | online brand | 0.0931 |
brand value | 0.1514 | brand experience | 0.0804 |
brand relationship | 0.1291 | Co-brand | 0.0799 |
5 Corrupting Brands, Consumer Loyalty and Supply Chains
Section 4 discovers four clusters of consumer and brand loyalty programs and their unique enabling techniques. These scopes are (C1) Customer Transaction Business Intelligence Analysis, (C2) Device/Platform Apps and UIs, (C3) Customer Relationship Management, and (C4) Brand Equity Evaluation. With the growing popularity of these consumer loyalty implementations, they are common targets for unethical use with potential disruption. In Table 5 ~ Table 8, we present examples of unethical or illegal practices in each cluster, which require strategic attentions to prevent the disruption of consumer loyalty and trusts in global food supply chain. The ontology of the four areas of consumer and brand loyalty programs and their typical unethical or illegal acts are shown in Figure 1.
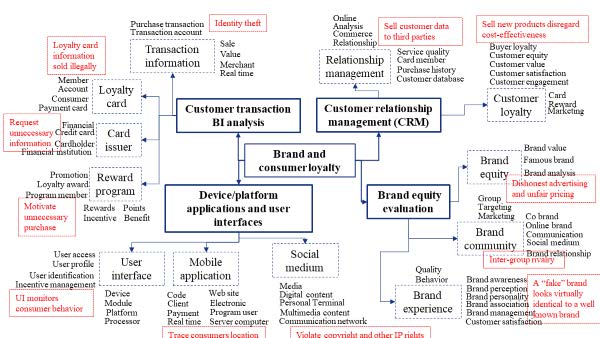
Figure 1. The ontology of consumer and brand loyalty programs and examples of disruptive acts (in red boxes).
Table 5: Unethical or illegal acts related to the scopes of customer transaction BI analysis.
Customer Trans BI | Unethical (or illegal) acts |
Transaction Information | Transaction information can be stollen from ATM’s, internet interaction with the food retailer or service provider, and of course, identity theft is an ongoing threat. Getting money at the supermarket or from isolated automatic tellers is not a risk-free activity. |
Card Issuer | Card issuers requested information may not be necessary. |
Reward Program | Reward programs are often a means to entice unhealthy overconsumption, the unnecessary purchase of goods to obtain a reward that is less valuable than the expensive of the purchases needed or services used. |
Loyalty Reward | Loyalty rewards are often tiered value cards (elite membership cards) that may have fees that do not justify their use. Of course, there is prestige value in holding certain loyalty cards or being allowed entry to the airport lounge to consume food and beverages before a flight. The question is the fairness to all customers who should be treated equally and fairly and the cost of the reward should be fairly valued which frequently is not worth the money. |
Member Loyalty | Membership loyalty can be a promotional campaign where the value of being a member is more beneficial in value to the provider than the customer. Five-year members may get a 5% discount on special items that may be marked up in price and are not a discount or special offer in exchange for their loyalty. |
Purchase Transaction | Purchase transactions can be monitored. The latest is the use of an application on the user’s cell phone that reads a bar code. New apps for unknown food stores appear on your phone in an attempt to lore you away from brands that you trust. A new food store should always be approached with suspicion and a lot of questions about country of origin and the supply chain. Brands need to be carefully inspected for infringement if you are in a new food sales environment. |
Loyalty Account | Loyalty accounts keep track of you and what you are buying and where you are going and have people calling you to ask you questions that you may find to be intrusive. A store may also use a push strategy to lower inventories of food items that are near their due date or not popular. |
Table 6: Unethical or illegal acts related to the development of apps and UIs.
APPs and UIs | Unethical (or illegal) acts |
User Account | User accounts can be stollen by phishing, embedded devices on ATMs, hacking into cloud servers, and a growing number of cons that trick the consumer into providing a thief with privileged account information. Sometimes, the user account information is stollen from the product or service provider which is corporate espionage. Be particularly careful of open market and street food market vendors with old credit card machines. |
Social Medium | Few consumers read the user agreements on the social medium that they use on their computers and cell phones. If I am using a social medium, what are the copyright and other ownership rights that I have to the content that I provide? Where is the data being stored and is my content being sold without authorization? |
User Interface | Every user interface can be a recorder. Your user interface monitors your consumer behavior and every interface is potentially a data collector that you have no knowledge of its functions. |
Mobile Application | A mobile application traces the consumers location. Your movements can also be linked to the people you meet which creates a network of your social world. Which vineyards you visit identifies your income. |
Digital Content | Digital content such as e-mail is read, and sorted, scanned for topics, linked to friends, stored in auxiliary databases without your control to edit or delete. |
Web Site | Web sites can be designed that look exactly like the web site that you use to do bank transactions or preform any online activity. Consumers must continuously be on the lookout for fake websites. |
User Profile | Your user profile is unknown to you and may be discriminatory. Attempting to verify your user profile in relations to others using computer applications and social media sites is a fruitless endeavor for most consumers. |
Alphanumeric Sequence | Applications can generate sequence numbers to identify themselves or can be generated to allow catching of transaction information. However, viruses can change the alphanumeric sequence on your computer or mobile application to substitute another app that may look the same as the app you are affiliated and steal information from your computing device. |
Real Time | Have you ever entered a website and started to fill in an application in real time only to receive a reply like “application timed out for inactivity?” Then you try and get back in and it does it again. Be careful, this is a good way to collect a lot of personal data. Everything from online voting applications to professional resumes and school applications use this unethical practice. There are many unethical real time data collectors online. |
Table 7: Unethical or illegal acts related to CRM.
CRM | Unethical (or illegal) acts |
Customer loyalty | Enterprises may abuse customer loyalty as they believe their customers will buy their new products regardless of usefulness, cost-effectiveness, or quality. Conspicuous consumption of food and beverages, particularly in today’s environment, is definitely viewed as unethical by the public and diminishes the standing of the brand and the consumer (https://www.amazon.com/Apple-MWUG2LL-A-Pro-Stand/dp/B082LQSZB8). |
Customer satisfaction | Some mobile phone application developers hire public relations companies to fake reviews on food store and merchandise assortment ratings as well as restaurants. The purpose of misrepresenting the ratings of customer satisfaction is to draw customers to a store that may be selling food items of extremely low quality, that are trademark infringement and not genuine, are have disguised sources of origin, selling homecooked, non-regulated food to the public, or may contain illegal ingredients (https://www.npr.org/sections/thesalt/2012/09/12/160755775/five-ways-to-spot-a-fake-online-review-restaurant-or-otherwise). |
Service quality | Genuine brand merchandise often comes with an augmented service such as replacement parts, discounts, and warranties that reinforce the quality of the brand. If you purchase a branded good from an unauthorized channel, you cannot receive a refund. |
Customer database | Selling customer data to third parties without customer consents. |
relationship quality | Exploiting emotions, evoking rage or sadness to manipulate consumer decisions |
Table 8: Unethical or illegal acts of cluster 4 – Brand equity evaluation.
Brand Equity | Unethical (or illegal) acts |
Brand equity | Dishonest advertising and unfair pricing are harmful to the customer’s brand attitude and lowers the brand equity. |
Brand community | A brand community deliberately provokes negative views of rival brands and their users. Using a sense of inter-group rivalry, an anti-brand community can denounce other brand by "trash talk" and “negative word-of-mouth”. |
Social medium | Use social media to get consumer-specific, intimate data. Based on data collected mainly on social networking sites, marketers and branders can know almost all of a person’s information-they can know information such as interpersonal relationships, dining habits, preferences, political opinions, religious beliefs, hobbies, and shopping history. |
Brand value | A brand deliberately lowered its brand value, intending to make foreign investment. |
Brand relationship | Unethical advertising creates many permanent stereotypes or encourages people to buy things that are not really needed. |
Famous brand | After gaining consumer trust and reputation, a brand secretly sells genuine and fake goods in turn, relying on consumer trust. |
Consumer brand | Brands that mislead, exploit or spam consumers is considered doing unethical marketing behavior. Unethical marketing practice may include intentionally evoking rage or sadness to manipulate consumer decisions, using fear tactics, targeting disadvantaged people or tricking customers into buying a product or service. |
Online brand | Since online marketers can collect numerous information about people's personal characteristics, online shopping behavior, and use of information, it is easy to invade individuals' privacy rights by selling information to other sources and divulging confidential information. |
Brand experience | When a store brand is packaged so that it looks virtually identical to a well know national brand. |
Co-brand | After a brand knows that it has a debt crisis, it intends to blame others' brands or cause others to fall into crisis by so-branding with other. |
6 Conclusions
This technology overview discovers the state-of-the-art R&D of customer and brand loyalty related technologies. Four dominant areas in R&D progresses are depicted (i.e., customer transaction BI analysis, devise platform applications and user interfaces, CRM, and brand equity assessment) through the collective intelligence of the past 20-year patents and NPL portfolio. After identifying the four key areas of customer and brand loyalty technology progress and implementation, the research emphasizes the critical challenges faced by the global food supply chain that could severely threaten and disrupt consumer and brand loyalty and destroy customer confidence. The systematic overviews of patents and NPL in the fields have provided a solid and reliable guidelines and technical solutions to avoid these disruptions. The research methodology and discovered outcomes are applicable to the consumer and brand protections for many industries that must value their consumer trusts and loyalty as the top priority. As we have identified, industrial ethics, such as in food, medicine and health case, are most relevant to the quality and well-being of our life. They must be well guarded and assessed through a systematic approach such as the continuous evaluation of brand and consumer loyalty.
Acknowledgement
This research is partially supported by the Taiwan Ministry of Science and Technology research funding (Project IDs: MOST-108-2410-H-009-025-MY2 and MOST 108-2221-E-007-075-MY3).
References
- Alpaydin, E. 2009. Introduction to machine learning, London: MIT press.
- Beck N. and Rygl, D. 2015. Categorization of multiple channel retailing in Multi-, Cross-, and Omni Channel Retailing for retailers and retailing. Journal of Retailing and Consumer Services, Vol. 27, pp. 170-178.
- Brynjolfsson, E., Hu, Y., Rahman, M.S. 2009. Battle of the retail channels: How product selection and geography drive cross-channel competition, Management Science, Vol. 55, Issue 11, pp.1755-1765.
- Executive Yuan, 2015. Productivity 4.0 to pilot industrial transformation, upgrade: Premier. Executive Yuan, Taiwan, Department of Information Services, https://english.ey.gov.tw/Page/61BF20C3E89B856/ef482c50-5a64-48d4-aa99-51d17843b1f1. Accessed July 2020.
- Hartigan, J.A., Wong, M.A. 1979. Algorithm AS 136: A K-Means Clustering Algorithm. Journal of the Royal Statistical Society. Series C (Applied Statistics), vol. 28(1), pp. 100-108.
- InnovationQ. 2020. Guide to InnovationQ: IP.Com Content and Coverage, Article: #310.
- Khan O., Greaves, Y.C. 2008. Mitigating supply chain risk through improved agility: Lessons from a UK retailer, International Journal of Agile Systems and Management, Vol. 3, Nos. 3/4, pp.263–281.
- Li, A.S., Trappey, A.J.C., Trappey, C.V., Fan, C.Y. 2019. E-discover state-of-the-art research trends of deep learning for computer vision. Proceedings, 2019 IEEE International Conference on Systems, Man, and Cybernetics (IEEE SMC 2019), Bari, Italy, Oct. 6-9.
- Lucas, A. 2020. Chick-fil-A no longer donates to controversial Christian charities after LGBTQ protests. https://www.cnbc.com/2019/11/18/chick-fil-a-drops-donations-to-christian-charities-after-lgbt-protests.html Accessed July 20, 2020.
- Nguyen, T. 2020. Boycotts show us what matters to Americans. Brand boycotts in the Trump era have been largely political, but with the pandemic comes a shift toward workers’ rights. https://www.vox.com/the-goods/2020/7/16/21325814/boycott-goya-pandemic-workers-rights. Accessed July 20, 2020.
- Prime Policy of Japan. 2013. New Growth Strategy: The Formulation of Japan Revitalization Strategy-Japan is Back. http://japan.kantei.go.jp/96_abe/documents/2013/1200485_7321.html. Accessed Oct. 10, 2016.
- Russell, S.J., Norvig, P. 1994. Artificial intelligence: A modern approach, Malaysia: Pearson Education Limited.
- Trappey, A.J.C., Trappey, C.V., Govindarajan, U.H., Chuang, A.C., Sun, J.J. 2017. A review of essential standards and patent landscapes for the Internet of Things: A key enabler for Industry 4.0. Advanced Engineering Informatics, Vol 33, pp 208-229.
- Verhoef, P.C., Kannan, P.K., Inman, J.J. 2015. From multi-channel retailing to Omni-channel retailing: introduction to the special issue on multi-channel retailing, Journal of Retailing, Vol. 91, Issue 2, pp. 174-181.
- Yan, Y.C. 2014. The third retail revolution: Embrace the era of consumer sovereignty, China machine press, China.